Improving Privacy Risk Detection with Sequence Labelling and Web Search
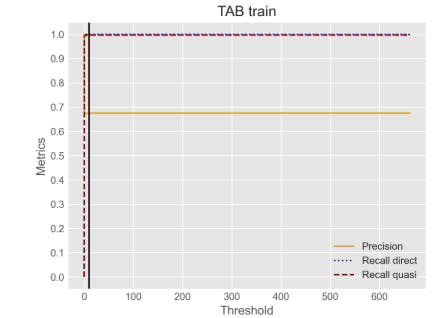
Authors:
(1) Anthi Papadopoulou, Language Technology Group, University of Oslo, Gaustadalleen 23B, 0373 Oslo, Norway and Corresponding author ([email protected]);
(2) Pierre Lison, Norwegian Computing Center, Gaustadalleen 23A, 0373 Oslo, Norway;
(3) Mark Anderson, Norwegian Computing Center, Gaustadalleen 23A, 0373 Oslo, Norway;
(4) Lilja Øvrelid, Language Technology Group, University of Oslo, Gaustadalleen 23B, 0373 Oslo, Norway;
(5) Ildiko Pilan, Language Technology Group, University of Oslo, Gaustadalleen 23B, 0373 Oslo, Norway.
TTable of Links
Abstract and 1 Introduction
2 Background
2.1 Definitions
2.2 NLP Approaches
2.3 Privacy-Preserving Data Publishing
2.4 Differential Privacy
3 Datasets and 3.1 Text Anonymization Benchmark (TAB)
3.2 Wikipedia Biographies
4 Privacy-oriented Entity Recognizer
4.1 Wikidata Properties
4.2 Silver Corpus and Model Fine-tuning
4.3 Evaluation
4.4 Label Disagreement
4.5 MISC Semantic Type
5 Privacy Risk Indicators
5.1 LLM Probabilities
5.2 Span Classification
5.3 Perturbations
5.4 Sequence Labelling and 5.5 Web Search
6 Analysis of Privacy Risk Indicators and 6.1 Evaluation Metrics
6.2 Experimental Results and 6.3 Discussion
6.4 Combination of Risk Indicators
7 Conclusions and Future Work
Declarations
References
Appendices
A. Human properties from Wikidata
B. Training parameters of entity recognizer
C. Label Agreement
D. LLM probabilities: base models
E. Training size and performance
F. Perturbation thresholds
5.4 Sequence Labelling
Yet another approach to indirectly assess the re-identification risk based on masking decisions from experts is to estimate a sequence labelling model. Compared to the previous methods, this method is the one that is most dependent on the availability of in-domain, labeled training data.
For this approach, we fine-tune a encoder-type language model on a token classification objective, each token being assigned to either MASK or NO MASK. For the Wikipedia biographies, we rely on a RoBERTa model (Liu et al., 2019), while we switch to a Longformer model (Beltagy et al., 2020) for TAB given the length of the court cases, as proposed in Pil´an et al. (2022). Due to discrepancies between the manually labeled spans or detected by the privacy-oriented entity recognizer, and the ones created by the fine-tuned model, we operate under two possible setups:
• Full match: We assume that a span constitutes a high re-identification risk if all of its tokens are marked as MASK by the fine-tuned Longformer/RoBERTa.
• Partial match: We consider that the span has a high risk if at least one token is marked as MASK by the Longformer/RoBERTa model.
5.5 Web Search
We used the Google API to query for each target individual in a given document and the unique text spans that occur in a given document[7]. The Google API provides 10 results per page. We limit the experiment to the top 20 results (i.e. first two pages from the web search). To avoid a prohibitively high number of API calls, we also constrain the search to individual text spans, although the same approach can in principle be extended to combinations of PII spans.
We also used the total number of hits reported by the Google search API for each PII span query. The assumption here is that if a search yields a larger number of responses, there is a higher chance that one of those responses will contain information about the target individual. However, generic search queries are also likely to return many responses. Therefore we considered applying an upper and lower bound on the total number hits. These thresholds were set experimentally to maximize the tokenlevel F1 scores on the TAB development set. This resulted in a lower limit of 100 hits and no upper limit. This method is limited by the potential unreliable nature of the total responses reported by web search engines, as shown in S´anchez et al. (2018).
[7] Web searches are from the period spanning July 2023 to September 2023.